
Modeling microRNA-target interaction network
Abstract
MicroRNA are short RNA molecules with an important role in post-transcriptional regulation of the gene expression. By now, approximately 2.000 microRNA have been identified, and each of them can regulate the expression of thousands of mRNA targets. Since the human genomes counts for approximately 20.000 mRNAs, we have to unravel a tight and complex biological interaction network, called mirnome. In addition, the scenario is complicated by the fact that each cellular tissue is characterized by a specific gene expression profile. As a consequence, the actual interaction network is tissue specific. In this project, we aim to model any tissue specific interaction network, focusing our studies on cancer tissues, in order to detect the anomalies in the interaction network with respect to the normal tissues’ behavior.
MicroRNA and mRNA expression profiles necessary to model the tissue specific interaction network can be obtained by high throughput data analysis techniques, based on microarray or NGS technologies.
These technologies provide quantitative information about all microRNAs and mRNAs endogenously expressed in the analyzed tissue. It is our aim to develop algorithms to model and compare the microRNA-target interaction network of tissues in different conditions.
Impatto:
Biological Big Data repositories are rapidly growing, partly due to the fact that in order to publish results in the most important journals, it is mandatory to make available to the public the original data used to obtain the results described on the paper. When data archives for gene expression profiles are needed, researchers use data repositories as Gene Expression Omnibus or ArrayExpress. As a consequence, if a researcher is interested in specific cellular tissues, it is highly probable that such data repositories contain a huge collection of suitable set of gene expression profiles. This kind of data contains the information of the expression of the entire genome in the tissues of interest and it is generally useful to perform the initial screening to decide on which features focus the research. In the face of a huge amount of available data, what is missing is data analysis algorithms useful to integrate many sources of biological big data. While it is common practice to detect differentially expressed microRNAs or mRNAs among two different tissue conditions in order to detect anomalies in the expression profiles, it doesn’t still exist an established method to detect which of these anomalies affect the interactions among microRNAs and mRNAs. We aim to develop such methods, in order to bring new instruments useful to understand cancer causes, moving from asking “which genes are involved” to the more functional question “which interaction are affected”.
Pipeline
-
CLINICAL
NEED -
DISEASES
ANALYSIS - DISCOVERY
-
PRECLINICAL
VALIDATION -
PRECLINICAL
DEVELOPMENT -
CLINICAL
STUDIES
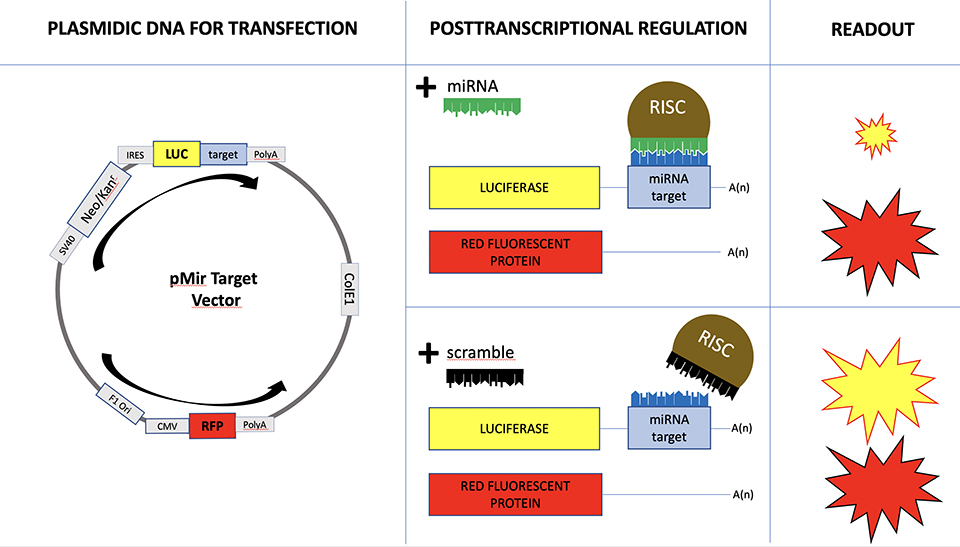
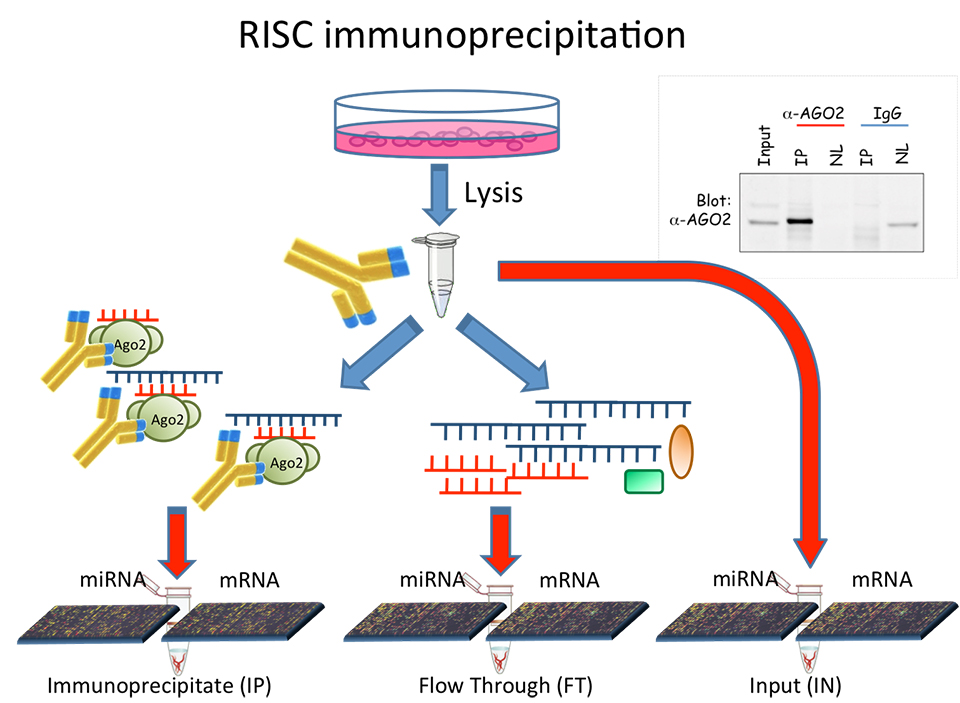
Principal Investigator
Contact
Therapeutic Areas:
Product:
Biomarkers, Biologics
Collaborations:
- Istituto per la Ricerca e l’Innovazione Biomedica (IRIB) – CNR, Palermo, Italia
- Dipartimento di Scienze e Tecnologie Biologiche Chimiche e Farmaceutiche (STEBICEF) – UNIPA, Palermo, Italia
- Dipartimento di Scienze Economiche, Aziendali e Statistiche (SEAS) – UNIPA, Palermo, Italia
- Dipartimento di Biologia Computazionale (CSB) – University of Pittsburgh, Pittsburgh, Stati Uniti
Scarica il pdf del progetto